Emerj is synonymous with enterprise AI access
Stay ahead of the machine learning curve with our newsletter and latest coverage.
Vendors: Reach Tech Leaders Enterprise Leaders: Subscribe for InsightsTechnology ROI Insights for Leaders
Join over 30,000 enterprise leaders who receive Emerj’s Tuesday AI ROI newsletter:
Emerj subscribers include AI adopters and champions within many of the world’s most respected brands:
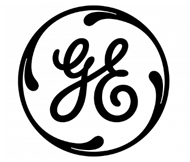
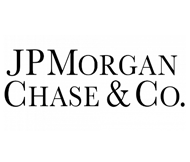
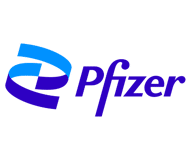
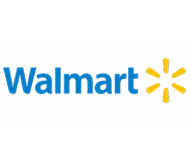
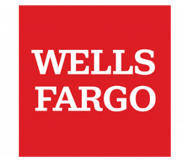
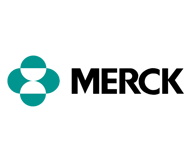